Put simply, data is the lifeblood of AI, data science, and analysis. Unfortunately, product data is rarely as clean as it needs to be. Anyone who has worked in data science and analytics, or AI and machine learning, will admit that they’ve seen their fair share of:
- Missing data that is incomplete and has holes;
- Messy data that is unstructured and lacks organization and standardization;
- Dirty data, the worst of the three, includes inaccurate information that must be corrected.
Poor data has wide-ranging impacts, from hindering analytical and modeling precision to ineffective personalization and increased consumer confusion and dissatisfaction, leading to poor customer experiences, lost sales, returns, and other negative impacts on retailers. It’s garbage in, garbage out.
Why Clean Product Attribution Data Is Critical
In retail, product attribution data is a critical input for many retail applications across merchandising, marketing, and e-commerce. Product attributes represent core information about each product’s details and benefits; the granular data that describes every aspect of the product. From objective attributes like type, fabric, and color, to subjective attributes like occasions, styles, and trends, these attributes or tags are critical, foundational inputs for effective product analysis and automation.
Without quality product attribution data, it’s nearly impossible for retailers to effectively optimize search, target consumers with personalized content, understand patterns around both purchasing and churn, make relevant product recommendations, automate and optimize product and marketing copy, forecast demand, and so much more.
Yet for many retailers, product attribution data is often missing, messy, or dirty.
What Are the Gaps in Product Attribution Today?
The right data bridges gaps between merchants and consumers. Ensuring that your retail data is clean and highly detailed is the most effective way to bridge this gap. Several factors go into ensuring you have available (not missing) and usable (not messy or dirty) data.
There are several common errors at a retailer’s product attribution and tag levels. These include issues with:
- Completeness: With such vast product offerings, it’s very easy for retailers to be stretched thin and overlook attributing all products at a granular level. Empty fields and missing key attributes are persistent problems across retail catalogs.
- Consistency: Retailers may use several different terms and phrases to describe the exact same things. For example, dresses with the same types of straps may be tagged as either “spaghetti strap” and/or “skinny strap.” Sandals with the same features may be inconsistently tagged as “flip flops” and/or “thongs.” Merchandisers can also have different definitions of attributes, like the definition of an A-Line fit, for example, which can embody inherent nuance and subjectivity.
- Accuracy: Sometimes, product attributes simply aren’t accurate. The color orange may be labeled as red. Kitten heels may be labeled as flats.
- Precision: Sure a dress or skirt can be short or long, but there’s a difference between midi, tea-length, ankle-length, maxi, and floor length.
Beyond inaccuracies, product attributes are also frequently missing at categorical levels. Even if you’re very descriptive of the product itself, there are many properties to account for, in addition to physical appearance. For example, product attributes may be missing:
- Specific Styles: A furniture retailer’s wooden dining room table may “evoke feelings of the temperate Tuscan countryside.” But if it’s missing basic descriptive attributes of its “rustic style,” shoppers may never find it.
- Occasions: If you’re selling pastel sundresses, and don’t list them as the perfect Easter dresses, you’re missing opportunities to connect with consumers who are shopping for holiday outfits.
- Trends: The trend cycle is faster than ever, thanks to social media, and new TikTok-inspired trends seem to pop up weekly. AI ensures that retail data is consistently updated to include critical trends, no matter how new and micro they are.
For many retailers, the product data they have from descriptions or other sources is typically unstructured data, rather than structured data. This matters because clean data alone is only half the answer. It also needs to be structured in a highly organized, often tabular format, to be easily decipherable by machine learning algorithms. Unstructured data, on the other hand, is messy data that must be transformed into structured data to automatically extract value. Combine a dirty data problem with a messy data problem; then you’re back to garbage in, garbage out.
How Can You Ensure You’re Working With Clean Retail Data?
Clean data doesn’t happen without concerted, ongoing effort and investment.
Retail marketplace buyers and sellers can be particularly challenged to maintain clean data due to the third-party relationship and the scale of the product data that is managed and shared. However, retailers and brands of all sizes suffer similar issues, so everyone benefits by investing in product attribution enrichment solutions, whether they buy or build such systems in-house.
One of the many benefits of working with an attribution solution like Lily AI, who has enhanced the attribution for billions of fashion, home, and beauty products, is operationalizing an ongoing process to ensure your systems are being fed with clean, structured product data. A key step in the Lily AI product attribution process, where Lily AI ingests product details and images and generates product attribution and copy, is transforming unstructured data into structured data using Natural Language Processing.
Lily AI’s product attribution helps retailers and brands at item setup all the way through improving data long after items have hit stores. Lily AI can refresh your product data by consistently adding new details, such as trends.
First Stop: Clean Data; Next Stop: PIM & Beyond
All this is to say that feeding clean data into your product data management system or PIM—is ideal. Yet, retailers can’t achieve this through a simple import. Rather, clean data requires proper taxonomy mapping, which can be a lengthy and highly detail-oriented exercise. That is, unless you’re working with a partner like Lily AI, which specializes in product taxonomies.
Once your enriched product attribution is mapped and imported accurately into your PIM, you’re ready to feed this data to other systems, like merchandising, marketing, and e-commerce platforms, and start driving greater profitability and growth!
See How Clean Retail Data Bridges Consumer Gaps
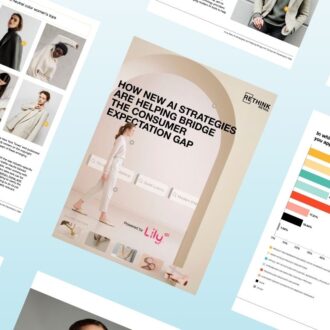