By Purva Gupta, Co-Founder and CEO of Lily AI
A recent article by Magna Ding in AI Secret dove into Microsoft’s latest research that introduces the concept of “Frontier Firms” — organizations that fundamentally restructure around hybrid teams of humans and AI agents working side by side.
The piece resonated with me on many levels, one of which is the necessity of managing expectations when it comes to the state of AI today, its accuracy, and the role that humans must still play in any impactful implementation.
In a Frontier Firm, AI is more than a tool — AI is serving as an active collaborator, capable of understanding context and providing nuanced recommendations that can transform decision-making and productivity. Of course, this is great! Yet garbage-in, garbage-out, as the old adage goes.
Garbage In, Garbage Out (GIGO)
The reality is that, when it comes to AI, the quality of an output is directly determined by the quality of the data it receives as input. If the data fed into the model is flawed — such as being inaccurate, incomplete, biased, outdated, or irrelevant — the resulting predictions, insights, or decisions produced by the AI will also be flawed or unreliable, no matter how advanced the underlying algorithms happen to be.
It’s surprising that many employees expect the AI capabilities to be 100% perfect out of the gate, serving as a panacea for ineffective work processes. They express disgust and dissatisfaction at the thought of having to play any role in overseeing the outputs or refining them for a particular business use. This is an unrealistic expectation; even OpenAI’s ChatGPT reminds its users that “ChatGPT can make mistakes. Check important info.”
Preparing for the Transition to Frontier Firms
While the idea of Frontier Firms is smart, and some version of it should be part of every organizational impact conversation, we shouldn’t overlook the potential psychological impacts that our employees are going through — many of our team members could be experiencing a stressful transformation themselves. Some want AI to do everything and anything for them, and some don’t want AI to do anything at all. The answer is somewhere in between, and the degree to which AI performs the work depends on the task at hand and the business objectives the AI is serving.
As we think about how we will leverage AI in our own organizations, we can do a better job of managing expectations with our teams, and clarifying the specific work of the human vs. the specific work of the AI. Generalized hybrid-work frameworks are certainly a good starting point, yet people who are actually doing the work need far more specificity and much deeper training.
AI Training Data Quality: A Team Effort
When it comes to uplifting our people and empowering them for the future we are already living in, we need to ensure that we provide them with the education necessary to understand their exact role in achieving quality AI outcomes. For instance, back to the data quality requirement, it is critical that we ensure our teams know that:
- AI models learn patterns from the data they are trained on; if that data contains errors or biases, the AI will replicate and even amplify those issues in its outputs.
- Poor data quality can lead to incomplete or outright incorrect results, undermining trust in AI systems and potentially causing real-world harm.
- High-quality data is essential for accurate, reliable, and fair AI performance. Ensuring data is accurate, complete, timely, and relevant is a foundational requirement for any successful AI implementation.
In this situation, we need to emphasize to our teams that “Garbage In, Garbage Out” is more than a basic principle for quality AI outputs; it serves as a warning that no matter how sophisticated the AI, its outputs can only be as good as the data it is given.
Training AI on Real, High-Quality Data
To bring this to life, here a few recent examples observed from various retailers. Anonymous, of course!
- Raw data input: one field shows country of origin as “Made in China” and another field shows the same product is “Made in Indonesia.” A different version of this data situation says “Made in China” vs. “MIITALY” for the same item.
- Raw data input: fabric content is reflected as “5% cashmere, 40% wool, 55% acrylic” yet human-entered copy says “100% cashmere.”
- Raw data input: care instructions say “Hand Wash” while another field says “Machine Washable.” Another version of this shows “Dry Clean Only” as well as “Machine Wash in Cold Water.”
- Raw data input: for the collar of a sweater, one field shows it as a “Stand Collar” and another field reflects “Turtleneck.”
- Raw data input: raw data shows that a top is reflected as “Slim Fit,” “Tailored Fit,” and “Relaxed Fit.”
- Raw data input: model is a “5’ 8” woman wearing a size small,” yet the detailed size specs were for a 6’ 2” male.
- Raw data input: Item “Runs Small” and also same item “Runs Large.”
The list goes on and on. In the above randomly selected examples, the data are being used to inform customer-facing product copy. Most of us would be quite disappointed if you thought you were purchasing a 100% cashmere sweater only to discover that over half of the sweater’s fabric content is acrylic when it arrives at my home.
As we experiment with AI in our own businesses, let’s bring our people along with us, helping them understand that we can only become a high-functioning Frontier Firm if our people better understand their critical role in ensuring quality AI benefits.
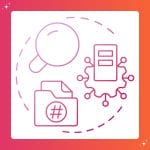